Leveraging AI for Demand Forecasting and Inventory Optimization Across Multiple Locations
In today’s dynamic business environment, companies face the daunting task of managing inventory across multiple locations while meeting fluctuating demand. Leveraging Artificial Intelligence (AI) for demand forecasting and inventory optimization can transform these challenges into opportunities for efficiency and growth. This article explores how AI technologies can revolutionize these critical aspects of supply chain management.
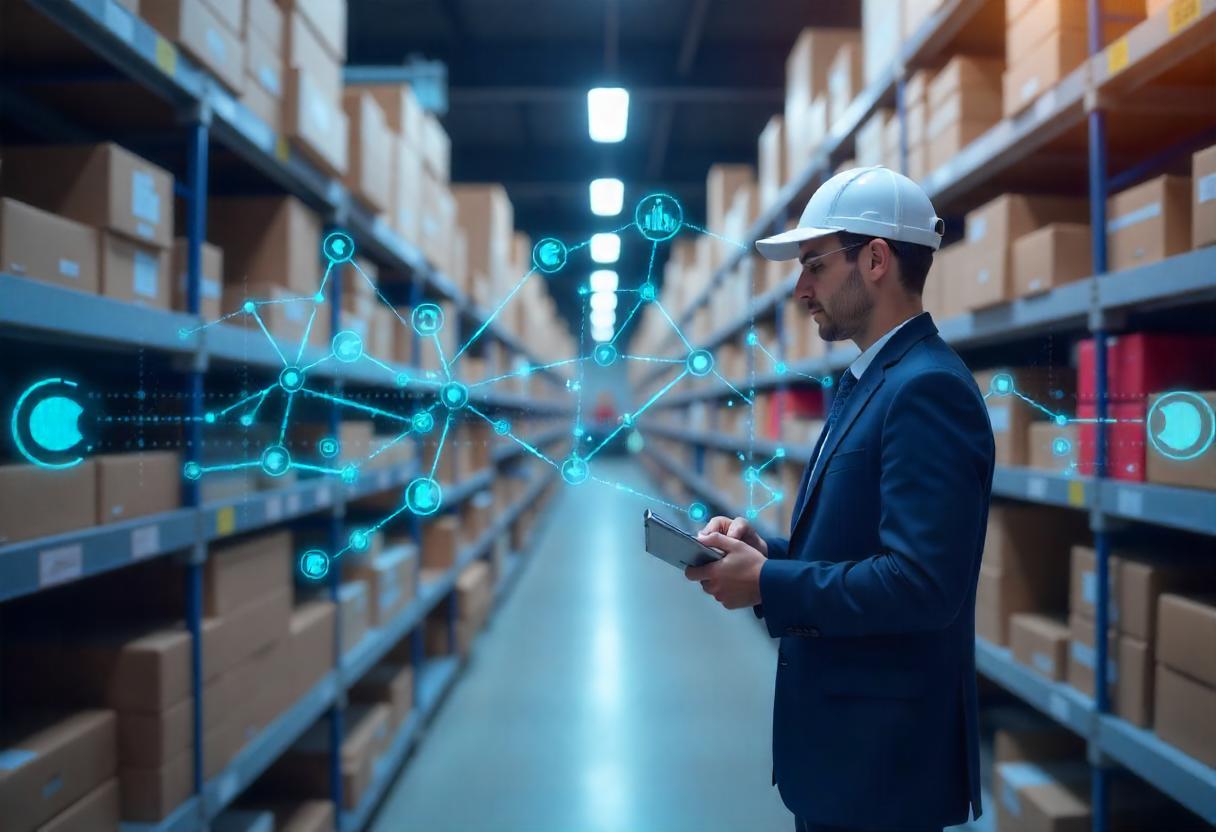
Introduction to AI in Demand Forecasting and Inventory Optimization
AI-driven demand forecasting and inventory optimization offer businesses a competitive edge by providing accurate, real-time insights. These technologies harness vast amounts of data to predict future demand and optimize inventory levels, reducing costs and enhancing customer satisfaction.
Key Concepts
- Demand Forecasting: Predicting future customer demand using historical data and advanced algorithms.
- Inventory Optimization: Ensuring the right amount of stock is available at the right location, minimizing excess and shortages.
The Role of AI in Demand Forecasting
Machine Learning Algorithms
Machine learning (ML) algorithms analyze historical sales data, market trends, and external factors (e.g., weather, economic indicators) to forecast demand with high accuracy. Techniques such as regression analysis, time series analysis, and neural networks are commonly used.
Example: Seasonal Demand Patterns – A retail chain with multiple stores can use ML algorithms to forecast seasonal demand for each location. By analyzing past sales data and considering local events and holidays, the AI system predicts the demand spike during the holiday season, allowing the company to stock up appropriately.
Natural Language Processing (NLP)
NLP can analyze text data from social media, news, and customer reviews to gauge market sentiment and predict demand shifts. This qualitative data complements quantitative sales data, providing a more comprehensive forecast.
Example: Social Media Trends – A fashion retailer uses NLP to monitor social media for emerging fashion trends. By identifying popular styles and predicting their impact on future sales, the company can adjust its inventory strategy to meet anticipated demand.
AI-Powered Inventory Optimization
Dynamic Inventory Balancing
AI can dynamically balance inventory across multiple locations by analyzing real-time sales data, supply chain logistics, and transportation costs. This ensures optimal stock levels at each location, reducing carrying costs and improving service levels.
Example: Warehouse Replenishment – A national distributor uses AI to manage inventory across its network of warehouses. The AI system continuously analyzes stock levels, sales data, and delivery schedules to recommend inventory transfers between warehouses, minimizing stockouts and excess inventory.
Predictive Analytics
Predictive analytics leverages AI to forecast inventory needs based on demand predictions. This proactive approach helps businesses prepare for demand fluctuations, avoiding last-minute rush orders and stock shortages.
Example: Production Planning – A manufacturer uses predictive analytics to forecast the demand for its products and adjust production schedules accordingly. By aligning production with anticipated demand, the company reduces excess inventory and minimizes the risk of stockouts.
Techniques for Implementing AI in Demand Forecasting and Inventory Optimization
Data Integration – Integrating data from various sources (e.g., sales, supply chain, market trends) is crucial for accurate AI predictions. Advanced data integration platforms and APIs facilitate seamless data exchange between systems.
Continuous Learning – AI systems should continuously learn from new data to improve forecasting accuracy. Implementing feedback loops and regular model updates ensures the AI adapts to changing market conditions and customer behavior.
Collaboration with Human Experts – AI augments human expertise rather than replacing it. Collaborating with supply chain professionals ensures that AI-driven insights are actionable and aligned with business goals.
Benefits of AI in Demand Forecasting and Inventory Optimization
Cost Reduction: By optimizing inventory levels and reducing excess stock, businesses can significantly lower carrying costs. Accurate demand forecasting minimizes rush orders and associated expenses.
Enhanced Customer Satisfaction: Meeting customer demand promptly improves service levels and customer satisfaction. AI-driven inventory optimization ensures products are available when and where customers need them.
Improved Decision-Making: AI provides data-driven insights that support strategic decision-making. Businesses can make informed choices about production, distribution, and inventory management, leading to better overall performance.
Implementation Strategies for AI in Supply Chain Management
Start with a Pilot Program – Implementing AI in a small segment of the business allows for testing and refinement. This approach helps identify potential issues and optimize the system before a full-scale rollout.
Invest in Training – Ensuring that staff understand and can effectively use AI tools is crucial for success. Regular training sessions and workshops can help employees become comfortable with new technologies and processes.
Leverage Cloud-Based Solutions – Cloud-based AI platforms offer scalability and flexibility. They allow businesses to access powerful computational resources and storage without significant upfront investment in infrastructure.
Future Trends in AI for Supply Chain Management
Integration with IoT
The Internet of Things (IoT) will play a significant role in the future of AI-driven supply chain management. IoT devices can provide real-time data on inventory levels, location, and condition, enhancing the accuracy of AI predictions.
Advanced Robotics
AI-powered robots will become increasingly prevalent in warehouses and distribution centers. These robots can perform tasks such as picking, packing, and sorting with high efficiency and accuracy, further optimizing inventory management.
Blockchain for Transparency
Blockchain technology can enhance transparency and traceability in the supply chain. When combined with AI, it can provide end-to-end visibility, ensuring that inventory data is accurate and tamper-proof.
Conclusion
Leveraging AI for demand forecasting and inventory optimization across multiple locations offers significant benefits for businesses. By adopting AI technologies, companies can enhance efficiency, reduce costs, and improve customer satisfaction. As AI continues to evolve, its applications in supply chain management will expand, providing even greater opportunities for businesses to thrive in a competitive marketplace.
By following these guidelines and implementing AI-driven strategies, businesses can transform their supply chain operations and achieve sustainable growth. Whether you are a retailer, manufacturer, or distributor, AI offers the tools you need to stay ahead in today’s dynamic market.